The prevalence and associated factors of underweight and overweight/obesity among adults in Kenya: evidence from a national cross-sectional community survey
Supa Pengpid, Karl Peltzer
Corresponding author: Karl Peltzer, Department of Research and Innovation, University of Limpopo, Turfloop, South Africa
Received: 08 Dec 2019 - Accepted: 14 Jan 2020 - Published: 25 Aug 2020
Domain: Chronic disease prevention
Keywords: Body weight status, health behaviour, health status, adults, Kenya
©Supa Pengpid et al. Pan African Medical Journal (ISSN: 1937-8688). This is an Open Access article distributed under the terms of the Creative Commons Attribution International 4.0 License (https://creativecommons.org/licenses/by/4.0/), which permits unrestricted use, distribution, and reproduction in any medium, provided the original work is properly cited.
Cite this article: Supa Pengpid et al. The prevalence and associated factors of underweight and overweight/obesity among adults in Kenya: evidence from a national cross-sectional community survey. Pan African Medical Journal. 2020;36:338. [doi: 10.11604/pamj.2020.36.338.21215]
Available online at: https://www.panafrican-med-journal.com/content/article/36/338/full
Research 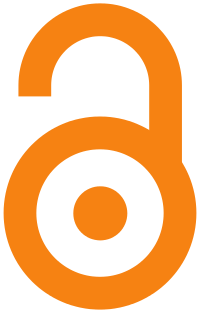
The prevalence and associated factors of underweight and overweight/obesity among adults in Kenya: evidence from a national cross-sectional community survey
The prevalence and associated factors of underweight and overweight/obesity among adults in Kenya: evidence from a national cross-sectional community survey
Supa Pengpid1,2, Karl Peltzer2,&
&Corresponding author
Introduction: the study aimed to investigate the prevalence and factors associated with underweight and overweight or obesity in an adult population in Kenya.
Methods: data from a cross-sectional nationally representative community-based study in Kenya (N=4283, 18-69 years) conducted in 2015 was utilized. Assessments included anthropometric, interview, blood pressure and biochemistry mesures. Multinomial logistic regression was used to assess the determinants of underweight and overweight or obesity relative to normal weight.
Results: in all, 11.9% of the study sample was underweight (BMI <18.5kg/m²), 60.1% had normal weight (BMI 18.5-24.9kg/m²), 18.9% overweight (25.0-29.9kg/m²) and 9.1% obesity (BMI ≥30.0kg/m²). In adjusted multinomial logistic regression, male sex (adjusted relative risk ratio-ARRR: 1.47, confidence interval-CI: 1.01, 2.13), lower education (ARRR: 0.63, CI: 0.46, 0.88), lower wealth status (ARRR: 0.47, CI: 0.29, 0.78), inadequate fruit and vegetable consumption (ARRR: 1.79, CI: 1.19, 2.70), adding daily sugar into beverages (ARRR: 1.49, CI: 1.01, 2.22) and having no hypertension (ARRR: 0.54, CI: 0.40, 0.74) were associated with underweight. Factors associated with overweight or obesity were middle and older age (ARRR: 2.15, CI: 1.46, 3.80), being female (ARRR: 0.30, CI: 0.22, 0.41), higher education (ARRR: 1.61, CI: 1.26, 2.24), greater wealth (ARRR: 2.38, CI: 1.41, 3.50), being a Kikuyu by ethnic group (ARRR: 1.68, CI: 1.19, 2.37), urban residence (ARRR: 1.45, CI: 1.06, 1.99), no current tobacco use (ARRR: 0.39, CI: 0.24, 0.54), low physical activity (ARRR: 1.49, CI: 1.02, 2.18) and having hypertension (ARRR: 1.96, CI: 1.54, 2.50).
Conclusion: more than one in ten were underweight and almost three in ten were overweight or obese among adults in Kenya. Several risk factors, including sociodemographic, lifestyle and health status risk variables, were identified for underweight and overweight or obesity, which can assist in developing intervention strategies targeting both these conditions.
Worldwide, among adults, 8.8% of men and 9.7% of women are underweight (Body Mass Index=BMI 18.5kg/m²) and 10.8% of men and 14.9% of women are obese (BMI ≥30 kg/m²) [1]. In the 2014 Kenya “Demographic and Health Survey”, 20.5% and 9.1% of women were overweight and had obesity, respectively [2]. Among adults (40-60 years) in urban slums of Nairobi, 19.6% and 5.1% of men and 30.9% and 32.1% were overweight and had obesity, respectively [3]. There is a dearth of recent national information among men and women on the prevalence and associated factors of underweight and overweight and obesity in Kenya. In Botswana 19.5% of men and 10.1% of women were underweight [4], 23% and 15% of women and 13% and 3% of men were overweight and had obesity, respectively [5], 7.5% were underweight and 31% overweight or obesity among women in Ghana [6] and 27.2% had obesity in South Africa [7]. Moreover, among adults, 6.5% were underweight and 21.9% had overweight and/or obesity in Malawi [8], 5.0% were underweight, 31% overweight and 17% had obesity among men and women in five urban areas in Nigeria [9] and 14.1% were underweight, 16.3% overweight and 4.3% had obesity among women in Addis Ababa in Ethiopia [10].
Having underweight in adulthood can have various negative health effects, including death [11], and having obesity has been associated with different non-communicable diseases (NCDs), including type 2 diabetes and cardiovascular disease, increasing mortality [12]. As reviewed in Pengpid et al. [13] factors associated with underweight in adulthood may include male sex, younger or older age, lower socioeconomic status, rural residence, health risk behaviours, such as smoking and insufficient food intake and fear of being obese. Sociodemographic factors associated with overweight or obesity include middle aged [2,14], female sex [8,14], higher socio-economic status [2,14,15], urban residence [2,8,14-16] and ethnicity, being Kikuyu [3]. Dietary risk behaviours impacting on obesity include the “consumption of energy-dense foods high in sugars and fat” [17,18] and insufficient fruit and vegetable intake [19]. Engaging in physical activity [20,21], khat [22] and tobacco use [8,17,18] have been found to decrease the likelihood of having overweight and obesity. Obesity is linked with several chronic NCDs such as hypertension and type 2 diabetes [3,23,24]. Kenya has a high proportion of under-nutrition in children (<5 years) (stunting 26% in 2014) [25], which may negatively affect weight status and NCDs in adulthood. The study aimed to investigate the prevalence and factors associated with underweight and overweight or obesity in an adult population in Kenya.
Data, study design and sample: in Kenya STEPS survey (2015) 4283 adults (18-69 years) were selected using multi-stage cluster sampling; detailed methods are described elsewhere [26]. The response rate ranged from 93% for biochemical masurements, 95% for questionnaire and 99% for physical and anthropometric measurements [26]. Ethics clearance was obtained from the “Kenya Medical Research Institute´s Ethics Review Committee (SSC No. 2607)” and written informed consent was attained from participants.
Measures: following WHO STEPS methodology [27] three steps were taken: step 1: questionnaire interview; step 2: anthropometric and BP measurements; and step 3: biochemistry tests. For step 1, handheld devices loaded with eSTEPS software and WHO STEPS questionnaire were used by trained data collectors at respondents´ residences [27]. Dietary behaviour was assessed with the following questions: consumption of processed food high in sugar (biscuits, wafers, cakes, candy, sweets and chocolate). Responses ranged from 1=always (every meal) to 5=never; consumption of soft drinks (like fanta, coca-cola, 7-up, aya, softa, vimto or other sugary drinks), (responses: number of days in a week and number of servings in one day); adding sugar to your beverages? (Responses ranged from 1=always (every drink) to 5=never); how often do you add salt or a salty sauce such as soya sauce to your food? (Responses ranged from 1=always (every meal) to 5=never); what type of oil or fat is most often used for meal preparation in your household? (Responses ranged from 1=liquid vegetable oil to 10=none used); daily intake of fruit and vegetables (FAV) were calculated from the number of servings consumed per day in a typical week. Insufficient FAV intake was defined as “less than five servings a day” [26]. Physical activity was classified as low, moderate and high, following guidelines from the WHO global physical activity questionnaire [28].
Current tobacco use was measured with two questions, “do you currently smoke any tobacco products, such as cigarettes, hand-rolled, cigars, water pipes/shisha or pipes/kiko” and “do you currently use any smokeless tobacco products such as snuff, chewing tobacco, kuber pan” (yes, no) [26]. Khat use was measured with the item, “do you currently chew Khat” (yes, no) [26]. Binge drinking in the past month was defined as having had ≥6 standard alcoholic drinks in a single drinking session [26]. Body Mass Index (BMI) was measured from scientific body height and weight measures. “The height measurements were taken in centimetre by using a portable height measuring equipment using unique ultrasonic and infrared technology. Weight measurement was done in kilogram using a portable electronic weighting scale” [26]. Pregnant women were excluded. Body weight categories were “underweight (<18.5kg/m²), normal weight (≥18.5-24.9kg/m²), overweight (≥25-29.9kg/m²) and obesity (≥30 kg/m²)” [29]. Blood pressure (BP) was averaged from three times taken and raised BP was defined as “systolic BP ≥140mmHg and/or diastolic BP ≥90mmHg or where the participant is currently on antihypertensive medication” [30]. Diabetes was defined as “fasting plasma glucose levels ≥7.0mmol/L (126mg/dl); or using insulin or oral hypoglycaemic drugs; or having a history of diagnosis of diabetes” [31].
Data analysis: the sample is described with statistics on frequency and weighted prevalence of BMI and other variables. Differences in proportion were tested with Pearson chi-square tests. Multinomial logistic regression was used to assess the determinants of underweight and overweight/obesity (with normal body weight status as reference category). Multi-collinearity was checked; no variable exceeding a value of 1.8. Missing values (<5%) were excluded from the analysis. P <0.05 was considered significant. All statistical procedures were adjusted for complex sample design and conducted with “STATA software version 13.0 (Stata Corporation, College Station, TX, USA)”.
Sample and body mass index status characteristics: the sample comprised 4283 persons 18-69 years (females=58.9%; median age 39 years, IQR=22) from Kenya. In all, 11.9% of the study sample was underweight (BMI <18.5kg/m²), 60.1% had normal weight (BMI 18.5-24.9kg/m²), 18.9% overweight (25.0-29.9kg/m²) and 9.1% obesity (BMI ≥30.0kg/m²). The highest proportion of underweight was in the 60 to 69 years age group (19.9% and among men 23.4%) and the 18 to 29 years age group (12.4%), while the highest proportion of obesity was in the 45 to 59 years age group (15.8% and among women 21.2%). Table 1 and Table 2 show sample and BMI status characteristics. In bivariate analyses, BMI status was higher in the older aged, women, urban residence, higher education, greater wealth, being Kikuyu, using liquid vegetable oil for meal preparation, those who were physically inactive, having hypertension and type 2 diabetes. The body weight status was lower in current tobacco and khat users and the body weight status did not differ by dietary behaviour variables (intake of fruit and vegetables, processed food and items containing sugar) (Table 1 and Table 2).
Associations with the prevalence of underweight and overweight/obesity: factors independently associated with underweight were male sex (Adjusted Relative Risk Ratio-ARRR: 1.47, Confidence Interval-CI: 1.01, 2.13), lower education (ARRR: 0.63, CI: 0.46, 0.88), lower wealth status (ARRR: 0.47, CI: 0.29, 0.78), inadequate fruit and vegetable consumption (ARRR: 1.79, CI: 1.19, 2.70), adding daily sugar into beverages (ARRR: 1.49, CI: 1.01, 2.22) and having no hypertension (ARRR: 0.54, CI: 0.40, 0.74). Factors independently associated with overweight or obesity were middle and older age (ARRR: 2.15, CI: 1.46, 3.80), being female (ARRR: 0.30, CI: 0.22, 0.41), higher education (ARRR: 1.61, CI: 1.26, 2.24), greater wealth (ARRR: 2.38, CI: 1.41, 3.50), being a Kikuyu by ethnic group (ARRR: 1.68, CI: 1.19, 2.37), urban residence (ARRR: 1.45, CI: 1.06, 1.99), no current tobacco use (ARRR: 0.39, CI: 0.24, 0.54), low physical activity (ARRR: 1.49, CI: 1.02, 2.18) and having hypertension (ARRR: 1.96, CI: 1.54, 2.50) (Table 3).
In this nationally representative community-based adult survey in Kenya, the found prevalence of underweight (BMI <18.5kg/m²) was 11.9%, overweight (≥25.0 - 29.9kg/m²) 18.9% and obesity (BMI ≥30.0kg/m²) 9.1%. These findings show a dual burden of underweight (11.9%) and overweight/obesity (≥25kg/m²) (28.0%) in Kenya. Similar figures for overweight and obesity were found among women in reproductive age in 2014 in Kenya [2] and for men in urban slums in Nairobi [3]. The prevalence of underweight was in this study among 40-60 year olds urban dwellers (14.8% among men and 7.8% among women) higher than in urban slums in Nairobi (11.7% among men and 3.9% among women), while the prevalence of obesity was higher among female urban slum dwellers in Nairobi (32.1%) [3] than among 40-60 year old female urban dwellers in this study (6.9%) (analysis not shown). The prevalence of underweight in this study in Kenya (14.3% among men and 9.5% among women) compares with the global prevalence among women (9.7%) but is higher in men (8.8%) [1] and compares with prevalence rates in Botswana (19.5% of males and 10.1% of females) [4] and Ghana (7.5% among women) [6] but was higher than in Malawi (6.5%) [8] and in urban areas in Nigeria (5.0%) [9] and was lower than among women in Addis Ababa in Ethiopia (14.1%) [10]. The prevalence of overweight or obesity (≥25kg/m²) (4.4% among men and 13.8% among women) in this study in Kenya was for men lower and for women similar to the global prevalence of obesity (10.8% in men and 14.9% in women), but similar to Botswana (3% in men and 15% in women) [5], higher than in Malawi (2.0% in men, 7.4% in women) [8] and lower than in South Africa (27.2%) [7].
The study found that the prevalence of underweight was the highest among 60-69 year-olds (19.9% overall, 23.4% in males and 12.8% in females) and young adults (18-29 years) (12.4%). Previous investigations also found a higher prevalence of underweight among older adults [14,32] and younger adults [32]. In this study, the prevalence of underweight was significantly higher among men than women, which is consistent with some previous studies in Africa [3,4]. In agreement with previous studies [4,14,15,32-34], this study found that the odds for undernutrition decreased with higher education and higher economic background status. Inadequate fruit and vegetable consumption was correlated with underweight, which may be indicative of food insecurity contributing to undernutrition. Consistent with a previous study [35], this study found that having hypertension decreased the odds of having underweight. In terms of overweight or obesity, consistent with previous studies [2,3,8,14-16] this study found that being female, being middle and older aged, having higher educational and economic status, residing in urban areas and being a Kikuyu were associated with having overweight or obesity. One possible reason for this could be that among the different ethnic groups in Kenya the largest epidemiological transition (regarding increase of overweight/obesity and decrease in underweight) has taken place among the Kikuyu in Kenya. Contrary to some previous studies [17-19], this study did not find an association between dietary behaviour and overweight or obesity. Consistent with previous studies [8,17,18,20,21], this study found that physical activity and tobacco use decreased the odds of having overweight and obesity. The possible mechanisms by which cigarette smoking can reduce body weight has been described “by increasing energy expenditure and inhibiting the expected compensatory increase in caloric intake” [36]. The link between obesity and chronic conditions such as hypertension and in bivariate analysis type 2 diabetes was confirmed in this study [3,23,24].
Study limitations: the study was limited by its cross-sectional design and apart from blood chemistry and physical measurements all the other information was based on self-reporting, which could have biased responses.
The study found in a national cross-sectional study that more than one in ten participants were underweight and almost three in ten were overweight or obese among adults (18-69 years) in 2015 in Kenya. Several risk factors, including sociodemographic, lifestyle and health status risk variables, were identified for underweight and overweight or obesity, which can assist in developing intervention strategies targeting both these conditions.
What is known about this topic
- Overweight or obesity is increasing and underweight decreasing among adults in Africa;
- In the 2014 Kenya “Demographic and Health Survey”, 20.5% and 9.1% of women were overweight and had obesity, respectively.
What this study adds
- The current study showed that 11.9% were underweight (BMI <18.5kg/m²), 18.9% overweight (25.0-29.9kg/m²) and 9.1% obesity (BMI ≥30.0kg/m²);
- Male sex, lower education, lower wealth status, inadequate fruit and vegetable consumption, adding daily sugar into beverages and having no hypertension were associated with underweight;
- Middle and older age, being female, higher education, greater wealth, being a Kikuyu by ethnic group, urban residence, no current tobacco use, low physical activity and having hypertension were associated with overweight or obesity.
The authors declare no competing interests.
Supa Pengpid and Karl Peltzer designed and conducted the analysis, drafted and revised the paper. All the authors have read and agreed to the final manuscript.
The authors are grateful to the Kenya National Bureau of Statistics, which made the data on which this analysis was based available.
Table 1: sample and nutritional status by sociodemographic variables
Table 2: sample and nutritional status by health variables
Table 3: associations of independent variables with underweight and overweight or obesity (with normal weight as reference category)
- NCD Risk Factor Collaboration (NCD-RisC). Trends in adult body-mass index in 200 countries from 1975 to 2014: a pooled analysis of 1698 population-based measurement studies with 19.2 million participants. Lancet. 2016;387(10026): 1377-1396. PubMed | Google Scholar
- Mkuu RS, Epnere K, Chowdhury MAB. Prevalence and predictors of overweight and obesity among Kenyan women. Prev Chronic Dis. 2018;15: E44. PubMed
- Asiki G, Mohamed SF, Wambui D, Wainana C, Muthuri S, Ramsay M et al. Sociodemographic and behavioural factors associated with body mass index among men and women in Nairobi slums: AWI-Gen Project. Glob Health Action. 2018;11(sup2): 1470738. PubMed | Google Scholar
- Letamo G, Navaneetham K. Prevalence and determinants of adult under-nutrition in Botswana. PLoS One. 2014;9(7): e102675. PubMed | Google Scholar
- Letamo G. The prevalence of and factors associated with, overweight and obesity in Botswana. J Biosoc Sci. 2011;43(1): 75-84. PubMed | Google Scholar
- Doku DT, Neupane S. Double burden of malnutrition: increasing overweight and obesity and stall underweight trends among Ghanaian women. BMC Public Health. 2015;15: 670. PubMed | Google Scholar
- Sartorius B, Veerman LJ, Manyema M, Chola L, Hofman K. Determinants of obesity and associated population attributability, South Africa: empirical evidence from a national panel survey, 2008-2012. PLoS One. 2015;10(6): e0130218. PubMed | Google Scholar
- Msyamboza KP, Kathyola D, Dzowela T. Anthropometric measurements and prevalence of underweight, overweight and obesity in adult Malawians: nationwide population based NCD STEPS survey. Pan African Medical Journal. 2013;15: 108. PubMed | Google Scholar
- Okafor CI, Gezawa ID, Sabir AA, Raimi TH, Enang O. Obesity, overweight and underweight among urban Nigerians. Niger J Clin Pract. 2014;17(6): 743-9. PubMed | Google Scholar
- Tebekaw Y, Teller C, Colón-Ramos U. The burden of underweight and overweight among women in Addis Ababa, Ethiopia. BMC Public Health. 2014;14: 1126. PubMed | Google Scholar
- Nubé M, Van Den Boom GJ. Gender and adult undernutrition in developing countries. Ann Hum Biol. 2003;30(5): 520-537. PubMed | Google Scholar
- Pi-Sunyer X. The medical risks of obesity. Postgrad Med. 2009;121(6): 21-33. PubMed | Google Scholar
- Pengpid S, Vonglokham M, Kounnavong S, Sychareun V, Peltzer K. The prevalence and underweight and overweight/obesity and its correlates among adults in Laos: a cross-sectional national population-based survey, 2013. Eat Weight Disord. 2020 Apr;25(2): 265-273. PubMed | Google Scholar
- Biswas T, Garnett SP, Pervin S, Rawal LB. The prevalence of underweight, overweight and obesity in Bangladeshi adults: Data from a national survey. PLoS One. 2017;12(5): e0177395. PubMed | Google Scholar
- Pengpid S, Peltzer K. The prevalence of underweight, overweight and obesity and their related lifestyle factors in Indonesia, 2014- AIMS Public Health. 2017;4(6): 633-649. PubMed
- Delbiso TD, Rodriguez-Llanes JM, Altare C, Masquelier B, Guha-Sapir D. Health at the borders: Bayesian multilevel analysis of women's malnutrition determinants in Ethiopia. Glob Health Action. 2016;9: 30204. PubMed | Google Scholar
- Pengpid S, Peltzer K. Associations between behavioural risk factors and overweight and obesity among adults in population-based samples from 31 countries. Obes Res Clin Pract. 2017;11(2): 158-166. PubMed | Google Scholar
- Moon K, Krems C, Heuer T, Roth A, Hoffmann I. Predictors of BMI vary along the BMI range of German adults - results of the German National Nutrition Survey II. Obes Facts. 2017;10(1): 38-49. PubMed | Google Scholar
- Carnauba RA, Chaves DF, Baptistella AB, Paschoal V, Naves A, Buehler AM. Association between high consumption of phytochemical-rich foods and anthropometric measures: a systematic review. Int J Food Sci Nutr. 2017;68(2): 158-166. PubMed | Google Scholar
- World Health Organization (WHO). Fact sheet 311: obesity and overweight. World Health Organization. 2011. Google Scholar
- Hruby A, Manson JE, Qi L, Malik VS, Rimm EB, Sun Q et al. Determinants and consequences of obesity. Am J Public Health. 2016;106(9): 1656-62. PubMed | Google Scholar
- Alshagga MA, Alshawsh MA, Seyedan A, Alsalahi A, Pan Y, Mohankumar SK et al. Khat (Catha edulis) and obesity: a scoping review of animal and human studies. Ann Nutr Metab. 2016;69(3-4): 200-211. PubMed | Google Scholar
- Leggio M, Lombardi M, Caldarone E, Severi P, D'Emidio S, Armeni M et al. The relationship between obesity and hypertension: an updated comprehensive overview on vicious twins. Hypertens Res. 2017;40(12): 947-963. PubMed | Google Scholar
- DiBonaventura MD, Meincke H, Le Lay A, Fournier J, Bakker E, Ehrenreich A. Obesity in Mexico: prevalence, comorbidities, associations with patient outcomes and treatment experiences. Diabetes Metab Syndr Obes. 2017;11: 1-10. PubMed | Google Scholar
- World Bank. Prevalence of stunting, height for age (% of children under 5) - Kenya. World Bank Washington, DC. 2016.
- Ministry of Health, Kenya Bureau of National Statistics, World Health Organization. Kenya STEPwise survey for non communicable diseases risk factors 2015 report. Nairobi, Kenya: Ministry of Health. 2015.
- World Health Organization. STEPwise approach to noncommunicable disease risk factor surveillance (STEPS). World Health Organization. 2016.
- World Health Organization. Global physical activity questionnaire (GPAQ) analysis guide. Geneva: World Health Organization. 2012.
- World Health Organization (WHO). Obesity: preventing and managing the global epidemic - report of a WHO consultation. Geneva, Switzerland: WHO. 2000;894: i-xii, 1-253. PubMed | Google Scholar
- Chobanian AV, Bakris GL, Black HR, Cushman WC, Green LA, Izzo JL et al. Seventh report of the Joint National Committee of Prevention, Detection, Evaluation and Treatment of High Blood Pressure. Hypertension. 2003;42(6): 1206-1252. PubMed | Google Scholar
- NCD Risk Factor Collaboration (NCD-RisC). Worldwide trends in diabetes since 1980: a pooled analysis of 751 population-based studies with 4.4 million participants. Lancet. 2016;387(10027): 1513-30. PubMed | Google Scholar
- Siddiqui MZ, Donato R. Undernutrition among adults in India: the significance of individual-level and contextual factors impacting on the likelihood of underweight across sub-populations. Public Health Nutr. 2017;20(1): 130-141. PubMed | Google Scholar
- Ha do TP, Feskens EJ, Deurenberg P, Mai le B, Khan NC, Kok FJ. Nationwide shifts in the double burden of overweight and underweight in Vietnamese adults in 2000 and 2005: two national nutrition surveys. BMC Public Health. 2011;11: 62. PubMed | Google Scholar
- Hanandita W, Tampubolon G. The double burden of malnutrition in Indonesia: social determinants and geographical variations. SMM- Population Health. 2015;1: 16-25. PubMed | Google Scholar
- Méndez-Chacón E, Santamaría-Ulloa C, Rosero-Bixby L. Factors associated with hypertension prevalence, unawareness and treatment among Costa Rican elderly. BMC Public Health. 2008;8: 275. PubMed | Google Scholar
- Audrain-McGovern J, Benowitz NL. Cigarette smoking, nicotine and body weight. Clin Pharmacol Ther. 2011;90(1): 164-8. PubMed | Google Scholar